Don't Attack the Artist, Fix the Palette! And Give it a Break!
A seemingly inevitable concern troubled the AI image generation landscape. Google's new AI chatbot Gemini, otherwise used worldwide for its interactive, iterative, and problem-solving capabilities, faced worldwide criticism for generating images that perpetuated harmful stereotypes, often representing historical figures or events with a clear bias towards white individuals. This happened during February 2024 and understandably sparked a heated debate about the ethics and potential dangers of AI-generated visuals. There were strong remarks of Google's apathy and turning a blind's eye towards ethical AI in competition with Microsoft Copilot's Image Generation tool, which is already famous with its DALL:E capabilities.
How This Works
For most of us (like me, who only understand AI from managing a program or a project, not technically unless one of my Data Scientist takes all the pain to explain me!), let's imagine that Gemini (or for that matter DALL:E) AI image generator as a student with a massive library like the great Library of Congress in the US. at its fingertip and the student has a superpower to go through many books at a single time. This bright young student learns by understanding and internalizing countless images and the descriptions that accompany them. So, if the books mainly feature a certain type of person or depict events in a biased way, the AI will, unfortunately, internalize those same biases.
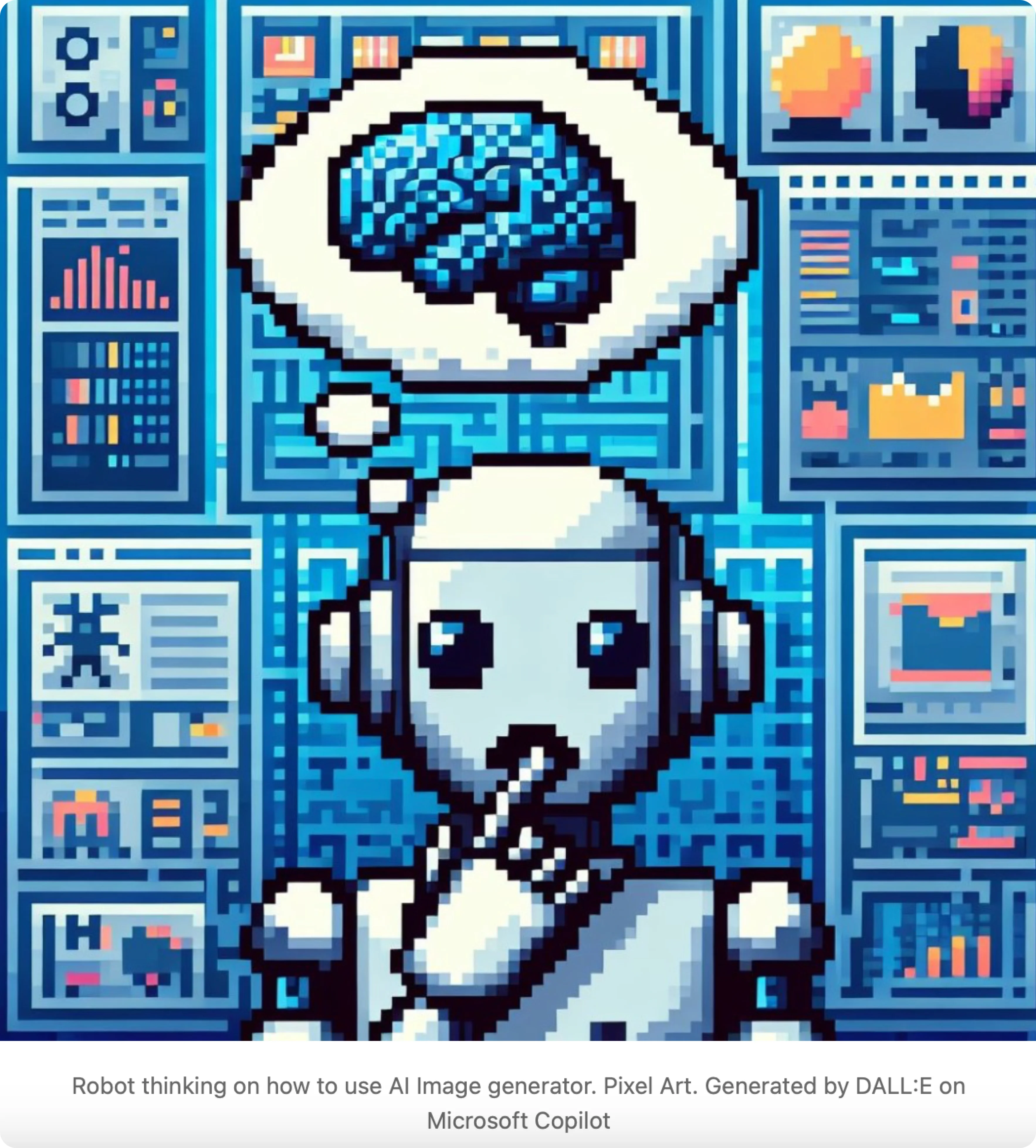
The Actual Problem
It's tempting and easy to blame the AI itself, to call it insensitive or malicious. Some even tried to misuse the tool to satisfy their perverse mindsets, manipulating prompts to bypass safeguards. But it's important to remember that AI is not an evil overlord. It's a tool that mirrors the data it learns from. Think of our library-loving student again. If they've only been exposed to books that present a skewed worldview, they can't magically produce unbiased images. Similarly, the AI can only reflect the data it's been fed. The issue lies in the inherent biases present within those massive datasets of images and text descriptions.
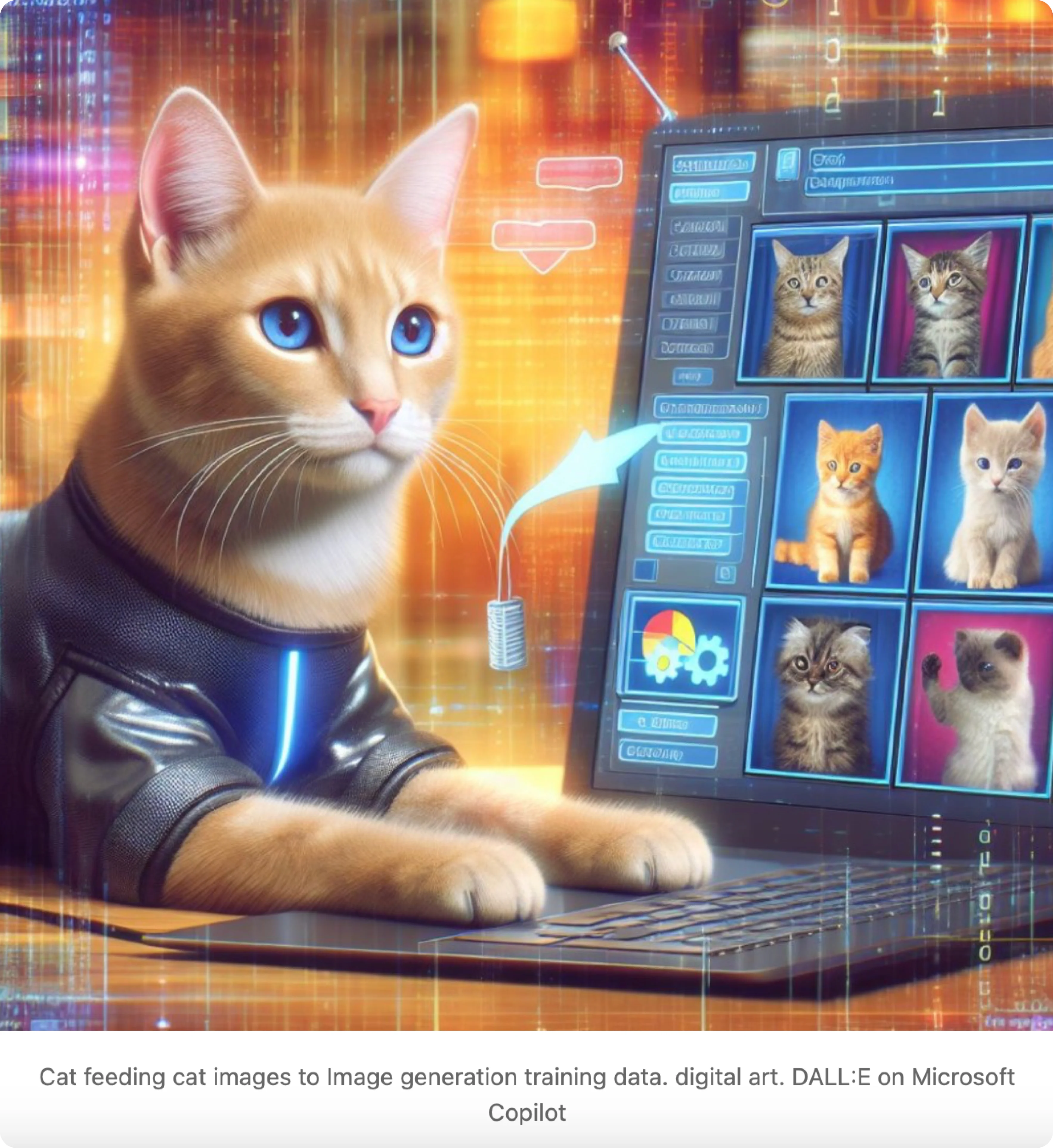
Who fed these images and how biases creep in?
Understanding where the AI's images come from is key to unravelling why biases exist in the first place. Here's a breakdown of the culprits:
- Dataset Creators: Companies and researchers compile massive image databases, and their choices on what to include or exclude can inadvertently introduce bias.
- Data Sources: Much data comes from the web, which reflects existing societal prejudices. Think of historical images, websites, or social media, where stereotypes and underrepresentation persist.
- Unconscious Bias: Even the most well-intentioned developers may unintentionally miss subtle bias patterns, leading to skewed datasets despite their best efforts. Examples of Common Biases are:
- Racial/Ethnic Bias: Overrepresentation of white individuals, underrepresentation of people of colour. Gender Bias: Stereotyping roles (e.g., women primarily in domestic settings, men in positions of power).
- Cultural Bias: Focus on Western history and events, lack of diverse cultural representation.
It's Widespread. Gemini isn't alone.
Gemini's struggles with bias highlight a systemic problem within AI image generation.
Unfortunately, this isn't an isolated incident. Let's look at other well-known image generators that have faced similar challenges:
- DALL-E (OpenAI): DALL-E has been known to perpetuate gender and racial stereotypes in its image outputs since many months. You can search with terms like "DALL-E gender bias examples" and you will see an article on Fast Company, describing how requests like "photo of a CEO" primarily generated images of men, while "photo of a flight attendant" were almost always women. And many more! (Search on Bing if you do not trust Google any more!). This article mentions how DALL-E often produces white faces as default when given racially ambiguous prompts. - here is an article from Washington Post and These are just a few examples how the biases are playing a role everywhere. Read on Washington Post
- Stable Diffusion: It is open-source and it definitely has become very popular among people adept at image creation with prompts. This offers some advantages; Stable Diffusion also reflects the biases of the vast and unregulated image datasets it's trained on. Read on Bloomberg.
- Midjourney: AI Image generation can struggle with representing diverse ethnicities. Midjourney often defaults to a European-centric appearance in historical or fantasy settings. Here is a comparative article in Forbes on how Gemini and its competitors can all be biased. Read on Forbes
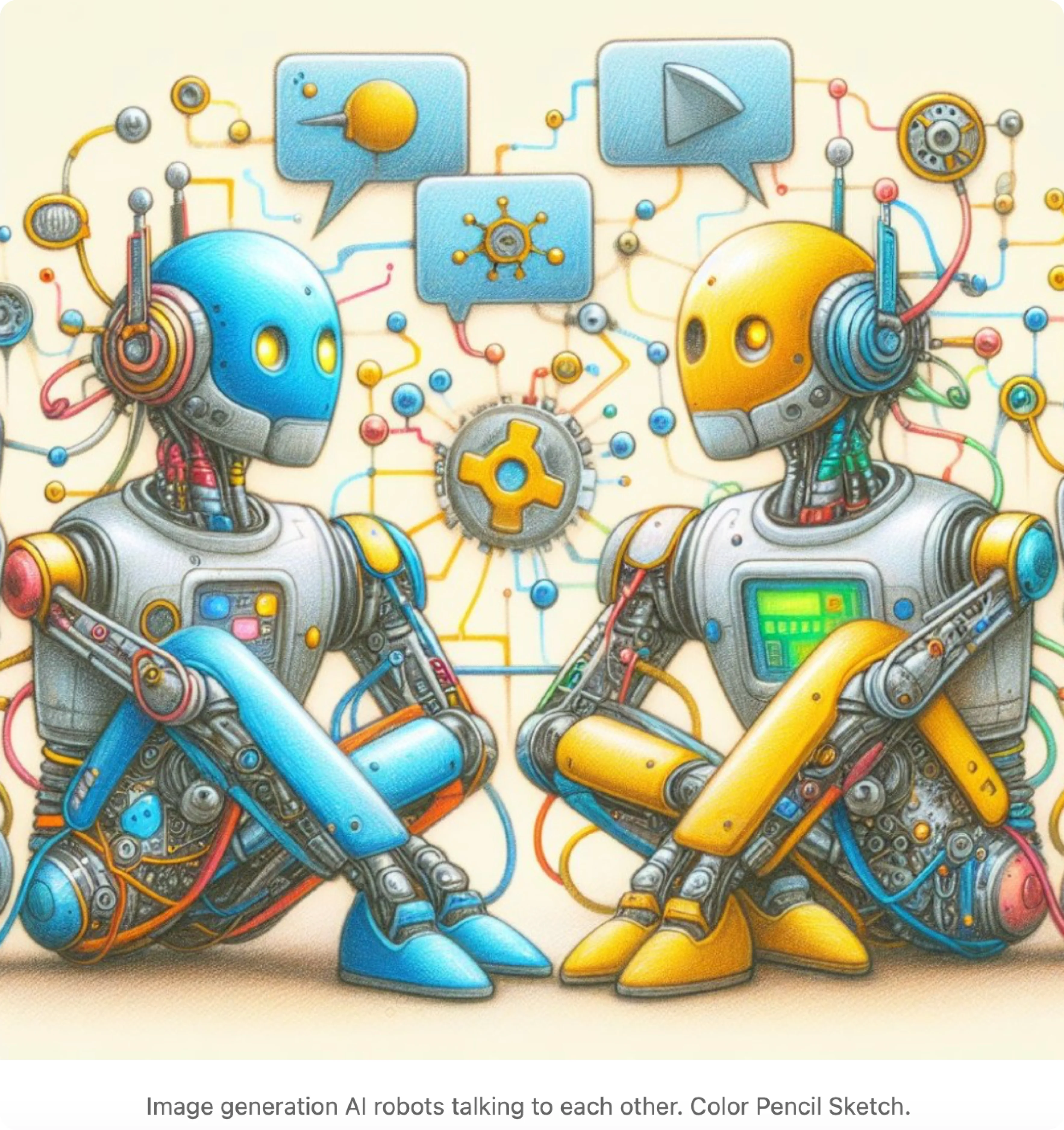
Here, the key point to emphasize is that no image generator is immune from biases and training data. And this proves blaming Gemini is not the solution, but re-defining the governance model and platforms to head on tackle this bias. It definitely requires industry-wide changes. This raises a crucial question: If these issues are so widespread, what responsibility do the strategists and creators of these AI tools have, and what actions can we demand?
Call to Action
Tackling bias in AI image generation demands a collaborative approach with a focus on shared responsibility. It's time for a Design Thinking approach for sustainable change. End users has to be critical consumers, recognizing and accepting that even advanced AI tools can reflect societal biases.
Developers and platform strategists will have to bear the pivotal accountability for proactive dataset auditing, implementing bias mitigation techniques (AI can take care if it too!) and prioritizing uncompromising transparency about their methods. There has to be consultation with experts across diverse fields – from ethics and social sciences to history and philosophy – to shape sustainable solutions.
Lastly (but never to end), everyone, from creators and users to the general public, deserves clear communication about AI capabilities, limitations, and potential risks. This includes responsible use guidelines and ongoing awareness campaigns to foster informed and ethical engagement with these powerful tools. Responsible use isn't solely on the providers of AI image generators; but falls on the users themselves.
The controversy around AI image bias is a reminder: technology mirrors us. The path towards more equitable AI starts with a more equitable society.
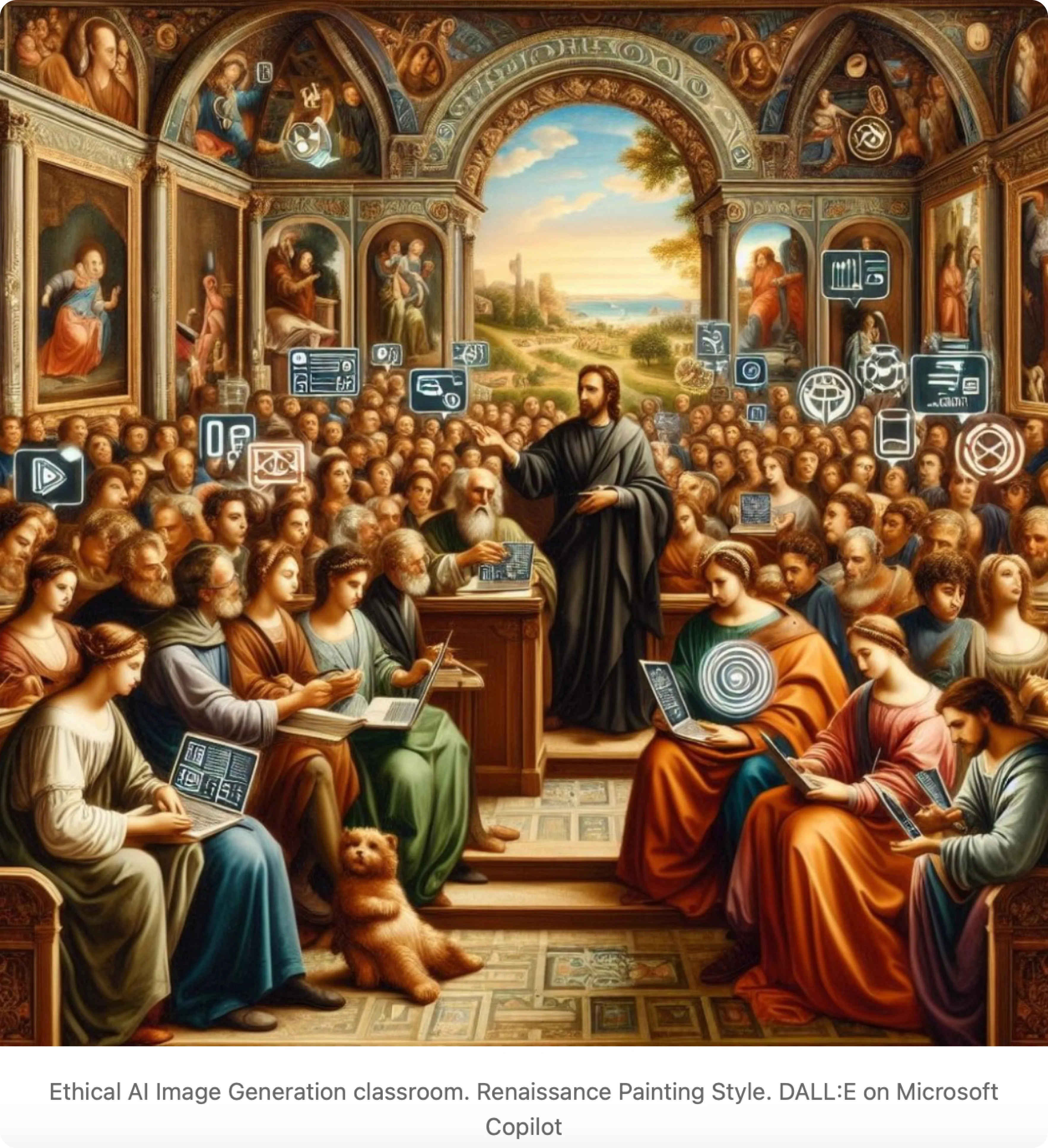
Read More
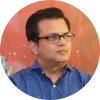
By Alok Ranjan
Project Manager
Read other blogs
Your go-to resource for IT knowledge. Explore our blog for practical advice and industry updates.
Discover valuable insights and expert advice.
Uncover valuable insights and stay ahead of the curve by subscribing to our newsletter.
Space Inventive | Powered by SpaceAI
Space Bot is typing